1. Introduction:
In today’s rapidly evolving business landscape, marketing optimisation has become an essential strategy for companies to stay competitive and achieve sustainable growth. The three pillars of marketing optimisation – customer high-value segmentation, personalised content, and ad spend allocation – play a pivotal role in maximising the effectiveness of marketing efforts. However, traditional marketing techniques have their limitations when it comes to these pillars. Fortunately, predictive analytics, when combined with external consumer behaviour data, has emerged as a game-changer, empowering businesses to overcome these challenges and supercharge their marketing optimisation strategies.
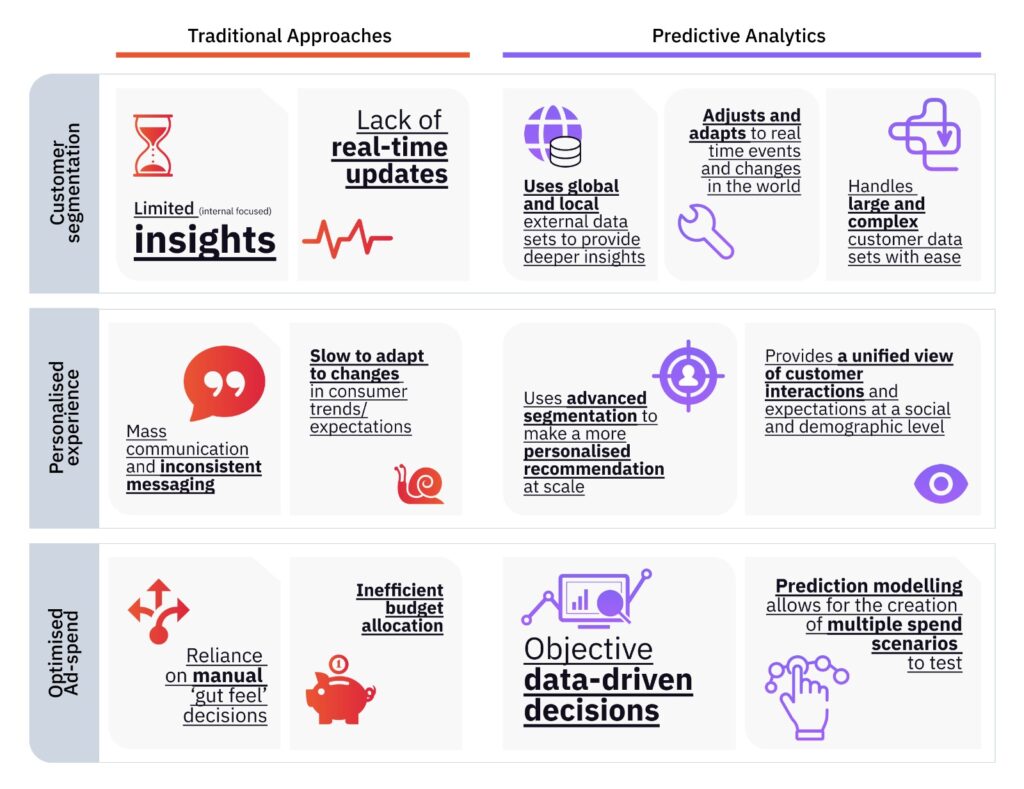
2. The Three Pillars of Marketing Optimisation:
2.1 Customer High-Value Segmentation:
Customer high-value segmentation involves dividing your customer base into distinct groups based on their value to your business. Traditionally, marketers rely on their own internal demographic data alone, leading to limited insights and an inability to handle complex customer data sets. Predictive analytics addresses these drawbacks by utilising advanced algorithms and machine learning techniques to analyse vast amounts of customer data from various sources. By integrating external consumer behaviour data, businesses gain a more comprehensive understanding of their customers’ preferences and behaviours beyond their own interactions.
Benefits of Predictive Analytics in Customer High-Value Segmentation:
- Identifying hidden patterns: Predictive analytics uncovers previously unknown segments of high-value customers based on external behaviour indicators that are relevant to the business.
- Real-time updates: Continuously analysing internal and external data provides real-time insights into customer behaviour, ensuring accurate and up-to-date high-value segments.
- Precision targeting: Predictive analytics refines the segmentation process, enabling more precise targeting of customers with the highest potential value based on both historical interactions and current behaviour patterns.
2.2 Personalised Content:
Personalised content involves delivering tailored marketing messages, offers, and experiences to individual customers or specific segments. Traditional marketing often relies on mass communication and manual customisation based on gut feel, leading to lower engagement and inconsistent messaging. Predictive analytics, combined with external consumer behaviour data, revolutionises personalised content creation and delivery.
Benefits of Predictive Analytics in Personalised Content:
- Advanced personalisation algorithms: Predictive analytics identifies patterns in external consumer behaviour. Allowing businesses to create more advanced personalisation algorithms and generate content recommendations based on consumers’ interactions with other brands and content types.
- Cross-platform consistency: By considering external consumer behaviour across different platforms, businesses maintain consistency in personalised content delivery regardless of where customers engage with the brand.
- Real-time content optimisation: Predictive analytics enables real-time optimisation of personalised content elements to meet consumers’ immediate needs and interests.
2.3 Ad Spend Allocation:
Ad spend allocation involves strategically distributing the advertising budget across different marketing channels and campaigns to maximise ROI. Traditional methods lack accurate measurement, limited real-time optimisation, and inefficient budget allocation. Predictive analytics, coupled with external consumer behaviour data, revolutionises ad spend allocation strategies.
Benefits of Predictive Analytics in Ad Spend Allocation:
- Enhanced attribution modelling: Predictive analytics attributes conversions and key metrics to specific advertising channels, considering both internal and external consumer behaviour data, allowing businesses to allocate ad spend to the most effective channels.
- External market trends: Analysing external consumer behaviour data helps businesses understand broader market trends and target emerging markets or new customer segments with high potential.
- Real-time optimisation: Predictive analytics provides real-time performance insights, enabling marketers to adjust ad spend allocation on the fly based on changing market conditions and consumer behaviour.
3. Conclusion:
The three pillars of marketing optimisation – customer high-value segmentation, personalised content, and ad spend allocation – form the backbone of successful marketing strategies. Traditional marketing techniques have their limitations, but predictive analytics, when combined with external consumer behaviour data, offers a powerful solution to overcome these challenges. By leveraging advanced algorithms, machine learning, and real-time insights, businesses gain a deeper understanding of their customers, create personalised and relevant content, and allocate their ad spend more strategically, ultimately leading to improved marketing performance and business growth in today’s dynamic market.